I have been working at the Southampton Education School for almost a decade and in that capacity deal with a lot of secondary datasets like PISA and TIMSS. One of the biggest challenging in analysing such datasets lies in the analytical choices that researchers make. To explore this so-called ‘analytical variability’, for the SCORE project, I replicated a gender finding for ICT literacy (Kim et al., 2014). It was my first attempt to have a completely transparent and open analysis ‘pipeline’ from raw data to result tables.
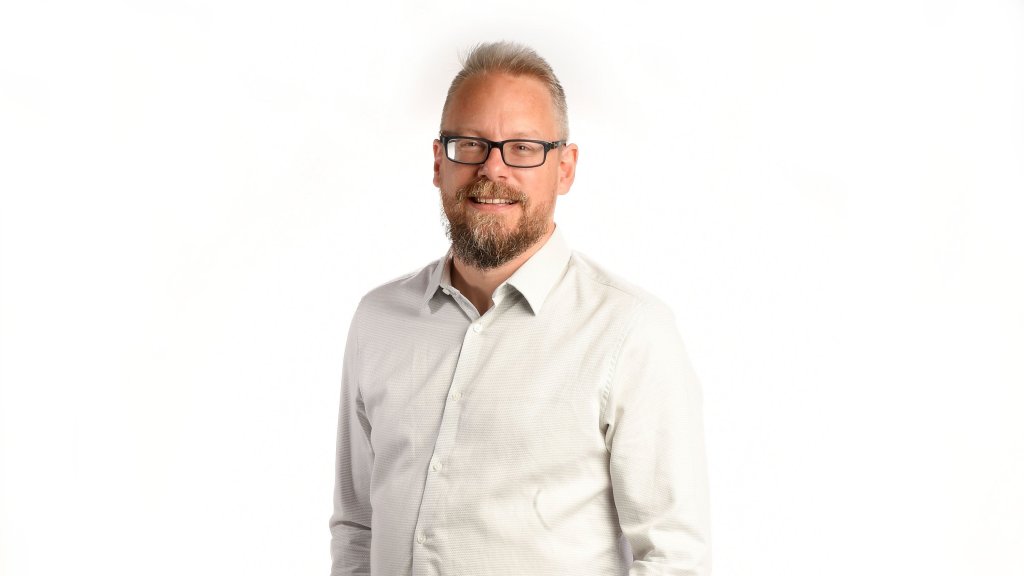
In the secondary data replication, I used data from the 2018 International Computer and Information Literacy Study, focussing on data from Korea as main replication. As many characteristics of the study as possible, such as variables and analytical strategy, were modelled in the analysis. Additional analyses included 13 countries and jurisdictions, varied centring techniques for variables, and missing data treatment. The replication and analyses were pre-registered via the Open Science Framework. The main analysis did not replicate the main gender finding. However, it was also clear that despite care taken in a rigorous replication, analytical variability still plays a large role in replications of findings, and with secondary datasets. I think the issue of analytical variability is relevant for almost all scientific disciplines, so this will have my attention for some time to come.